Drug fraud has been described by many as the perfect crime – a destabilising force on both the society and an individual's wellbeing. Recent advancements in analytical technologies could elevate quality control standards, potentially putting an end to this menace in both developed and developing countries. By James Lau Yi Wei
In 2012, the Food and Drug Administration (FDA) discovered Bevacizumab counterfeits being distributed to over 900 physicians across the USA, marking it as one of the most high-profile instances of counterfeit medications circulating within the American pharmaceutical supply chain. Bevacizumab is a targeted therapeutic drug used within traditional chemotherapy regimens. It works by inhibiting the action of Vascular Endothelial Growth Factor A (VEGF-A), halting the formation of new blood vessels around cancer tissues. Subsequent analytical testing revealed that these falsified formulations of Bevacizumab contained foodstuffs like corn starch and salt, whilst missing the active pharmaceutical ingredient (API) required to effectively treat cancer (Mackey et al., 2015).
A recent systematic review revealed that antibiotics, antimalarials, and anthelmintics represent the three most commonly encountered classes of substandard and falsified (SF) drugs (Tegegne et al., 2024). Besides causing treatment failure or adverse reactions in patients, SF drugs are drivers of antibiotic resistance and mortality rates (Cavany et al., 2023). Indeed, the case of counterfeit Bevacizumab accentuates the need for a robust regulatory system, even in well-developed nations. An inadequate response to this threat has the potential to erode public trust in key stakeholders and exacerbate poor health outcomes.
Light work: Near-Infrared Spectroscopy for SF Drug Detection
Near-infrared (NIR) spectroscopy is a versatile technology that could enhance national pharmacovigilance monitoring systems to combat SF drugs. Already used in the agricultural and food industry for products such as commercial honey (Qiu et al., 1999), NIR spectroscopy is extremely useful for analysing complex mixtures including pharmaceuticals. A light source emits radiation that interacts with the sample. This causes certain molecular bonds to absorb energy and undergo vibrational transitions. The remaining light, which does not get absorbed, is reflected and picked up by a detector to generate an absorption spectrum. As different functional groups (unique collections of bonds allowing SF detection) reflect or absorb light differently, the various absorption bands will correspond to the functional groups present. NIR is non-destructive, does not require sample preparation and generates results quickly (Pasquini, 2003). Given these advantages, NIR appears sufficiently capable for SF detection. However, its ability is limited by the requirement for massive reference libraries on the spectrophotometer, as variations in excipients (non-API ingredients) and APIs across brands and even batches of the same product can result in false positives. Ideally, manufacturers could cooperate with spectrophotometer brands to compile and update these libraries. However, this could be highly resource-intensive given the immense size of the pharmaceutical industry.
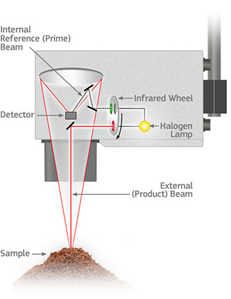
Figure 1: A diagram of a spectrophotometer used for rapid, non-destructive chemical analysis. A light source emits infrared light, directed onto the sample. The reflected light is captured by a detector and compared against an internal reference beam to determine the composition of the material. Adapted from Kpmanalytics.com (2024)
AI ‘Role’ Models Shaping Near-Infrared Spectroscopy
Machine learning has the potential to transform NIR Spectroscopy’s SF drug detection abilities. A study assessed this technology’s potential by testing six different machine-learning models which evaluated samples into “OK”, “SUB” and “FAIL” categories (Awotunde et al., 2022). These models were trained on and validated against 900 NIR spectra generated from pure paracetamol, lactose or ascorbic acid and binary mixtures of paracetamol with either substance. Whilst the models gave excellent accuracy with samples similar to the training data, they performed only moderately well with branded paracetamol products outside of the training data. To improve the accuracy of results using untrained data, all six models were combined into a predictive learning algorithm utilising a majority voting mechanism. Each model's classification contributed to the final decision, defaulting to “FAIL” in the case of no majority. Testing on trained and untrained data, including branded paracetamol and 20 other common pharmaceuticals, showed a staggering improvement in the results, as the algorithm correctly predicted the classification in 93% of samples.
From Bench to Field: Safeguarding the Pharmaceutical Supply Chain
Interestingly, technologies that identify SF drugs could be considered a public good due to their accessible and non-rivalrous nature. Consumers, pharmaceutical manufacturers and governments would all gain major benefits from the investment into such technologies and their implementation. These technologies could also mitigate other healthcare risks including the detection of novel psychoactive drugs like synthetic cannabinoids. In light of these considerations, government intervention in safeguarding public health is highly justified, especially when the private sector might be reluctant to invest into these technologies. This could be due to concerns over development costs, a lack of immediate return on investment and the likelihood of increased regulatory burdens and scrutiny on their own products.
One chief strategy for ensuring drug quality is to establish and maintain tight state control over the pharmaceutical supply chain (Hamilton et al., 2016). As such, incorporating such technologies into national pharmacovigilance schemes will strengthen state security and bolster consumer trust. Utilising a combination of both top-down and bottom-up approaches to identify SF drugs is crucial, as the effective detection of SF drugs is contingent on comprehensive policy making alongside effective deployment of technologies in the field. Unlike the provision of other traditional public goods, government intervention in the case of SF drugs requires a nuanced approach; there should be a fine balance between achieving public health imperatives, and a thorough consideration of private sector interests.
References
Cover image credit: Pexels
https://www.pexels.com/photo/medication-pills-isolated-on-yellow-background-3683096/
Awotunde O., Roseboom N., Cai J.,Hayes K., Rajane R., Chen R., Yusuf A., and Lieberman M. “Discrimination of Substandard and Falsified Formulations from Genuine Pharmaceuticals Using NIR Spectra and Machine Learning.” Analytical Chemistry 2022 94 (37),12586-12594.Availableat: https://pubs.acs.org/doi/10.1021/acs.analchem.2c00998?ref=pdf (Accessed: 3 November 2024).
Cavany, S., Nanyonga, S., Hauk, C., et al. (2023) 'The uncertain role of substandard and falsified medicines in the emergence and spread of antimicrobial resistance', Nature Communications, 14, 6153. Available at: https://doi.org/10.1038/s41467-023-41542-w (Accessed: 10 November 2024).
Hamilton, W. L., Doyle, C., Halliwell-Ewen, M., et al. (2016) 'Public health interventions to protect against falsified medicines: a systematic review of international, national and local policies', Health Policy and Planning, 31(10), pp. 1448–1466. Available at: https://doi.org/10.1093/heapol/czw062 (Accessed: 15 November 2024).
Mackey, T., Cuomo, R., Guerra, C., & Liang, B. (2015) 'After counterfeit Avastin – What have we learnt and what can be done?', Nature Reviews Clinical Oncology, 12(5), pp. 302–308. Available at: https://doi.org/10.1038/nrclinonc.2015.35 (Accessed: 8 November 2024).
Pasquini, C. (2003) 'Near Infrared Spectroscopy: fundamentals, practical aspects and analytical applications', Journal of the Brazilian Chemical Society, 14, pp. 10. Available at: https://www.researchgate.net/publication/26363879 (Accessed: 3 November 2024).
Qiu, P. Y., Ding, H. B., Tang, Y. K., & Xu, R. J. (1999) 'Determination of Chemical Composition of Commercial Honey by Near-Infrared Spectroscopy', Journal of Agricultural and Food Chemistry, 47(7), pp. 2760-2765. Available at: https://pubs.acs.org/doi/10.1021/jf9811368 (Accessed: 9 November 2024).
Tegegne, A. A., Feissa, A. B., Godena, G. H., et al. (2024) 'Substandard and falsified antimicrobials in selected East African countries: A systematic review', PLoS ONE, 19(1), e0295956. Available at: https://doi.org/10.1371/journal.pone.0295956 (Accessed: 2 November 2024).
In-text diagram:
kpmanalytics.com (2024). “Near Infrared Measurements - How do they work?”. [Accessed on: 10th February 2024]. Available from: https://www.kpmanalytics.com/blog/near-infrared-measurements-how-do-they-work