How to Train Your Fusion Reactor: Letting Tokamaks Learn Stability
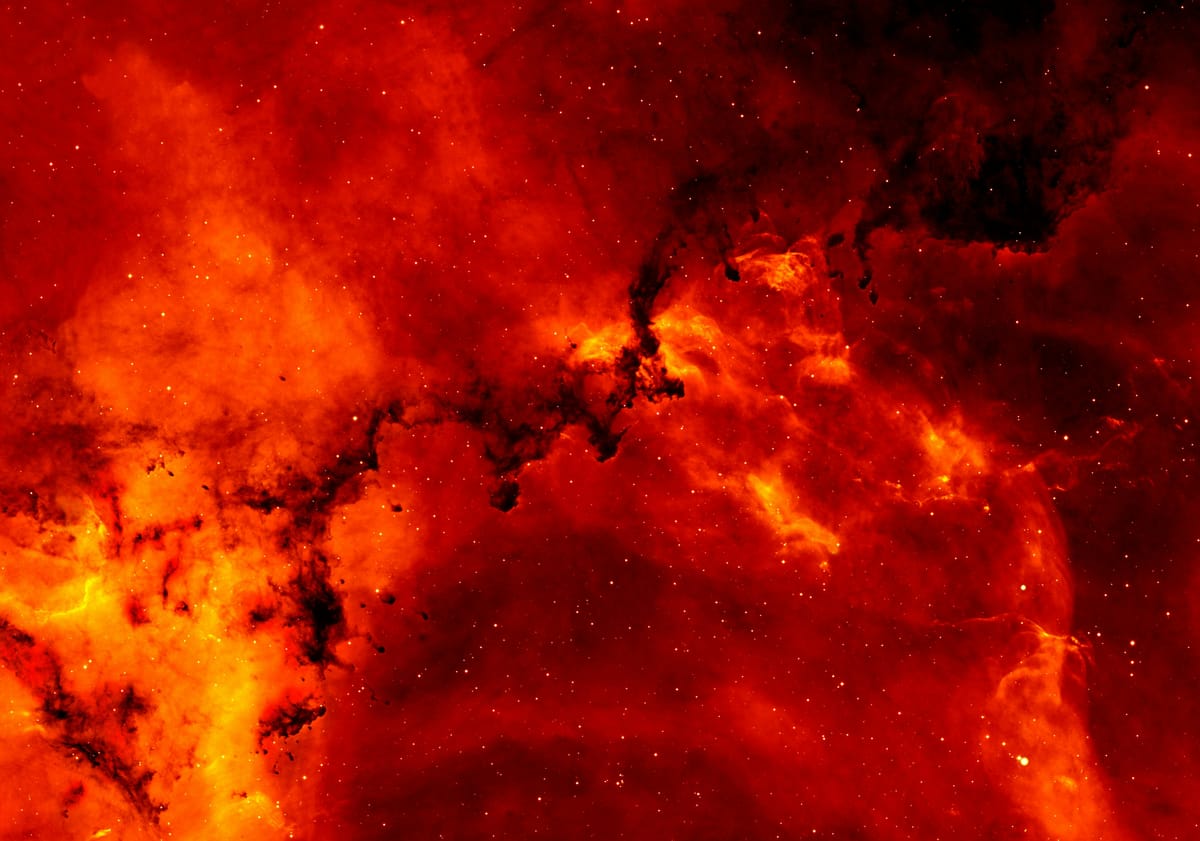
Fusion Reactors, while impressively useful for renewable energy production, often run into chaos due to plasma disruption events. A group of scientists tackled this problem using reinforcement learning, letting the reactor learn how to stay stable during operation. By Faraaz Akhtar
You may have heard of the notion that we are all stardust or that we come from what were once large balls of fire in the sky. Often, this is used to make us feel significant—on the same level as a star; as grand as one. The truth is, if you really dig into it, you’ll find that almost everything is stardust. When stars die, they release the elements they used to burn as fuel—elements that were forged in their cores through the power of nuclear fusion, the very process physicists are working to replicate here on Earth (Sagan, 1980).
Nuclear fusion is the process of fusing two particles together. It’s a process that releases a lot of energy. Moreover, the main by-product of fusion, helium, does not contribute to the greenhouse effect (Cardinal & Pizzuto, 2011). This means that if we could harness and utilise fusion, we would greatly help the problem of renewable energy. As such, it is currently a huge area of research, with labs all over the world hoping to be the first ones to be able to have a reliable way of getting net positive energy (ITER Organization).
In a nuclear reactor, a hydrogen fuel is heated to millions of degrees, turning it into a plasma. This plasma creates the necessary environment for light elements to ionise and fuse together, giving us energy. The plasma is contained using magnetic fields in a donut-shaped chamber called a tokamak; which ensures that temperatures don’t drop and that the plasma is kept stable (Wesson & Campbell, 2011). This is obviously easier said than done—trying to recreate the sun doesn’t exactly scream stability.
Reactors are often hit with what are called plasma disruption events—essentially a nuclear highway pile-up. The plasma becomes unstable and escapes from the tokamak reactor, which suddenly cools the plasma down, releasing a burst of energy that can be hard to control. At the same time, the electric current that helps keep the plasma steady also drops, making it even harder to contain. Runaway electrons speed up and damage the reactor walls (Hender et al., 2007).
It’s pandemonium.
And, so, in this desperate time, we turn to a familiar friend—machine learning. More specifically, reinforcement learning. Where we teach a machine what to do by giving it rewards and punishments. In a paper published in Nature earlier this year, a group of scientists employed reinforcement learning for the task of teaching a tokamak reactor to keep the plasma reaction stable (Seo et al., 2024).
To teach the reactor how to stabilise plasma, the reinforcement learning agent was first run using simulations. These simulations recreated the environment inside the DIII-D tokamak—the biggest reactor in the US. During training, the agent learned through trial and error—receiving a reward whenever it maintained stability and a punishment whenever it allowed a disruption. The agent gradually discovered patterns in how the plasma behaved, learning which adjustments to make to magnetic fields and control parameters to keep it contained. When it was used in the real DIII-D fusion reactor, it was able to contain the plasma well, even in unfavourable conditions of low torque: a slow rotation of the plasma. This has traditionally been difficult when the reactor is under pre-programmed control, and is an important development as the rotation of the plasma is one of the key factors that helps to stabilise it (Seo et al., 2024).
While a massive step forward, we do have to be cautious with such technology. It is certainly not a one-size-fits-all solution, and rigorous testing is required before applying it to newer and bigger reactors. As the Deputy Head of the Safety & Quality Department at the International Thermonuclear Experimental Reactor (ITER) Organization put it, “We need to…make sure that the technology is not only feasible, but that it is truly safe. Fusion is a new way to create energy, and is still very much a young technology.”(International Atomic Energy Agency (IAEA)., 2023). Still, with cautious optimism and excitement, we watch as stardust gives its best shot at recreating the stars.
References
Cover Image Credit - Pexels: https://www.pexels.com/photo/red-and-orange-solar-flare-73873/
Sagan, C. (1980) Cosmos. 1st edn. New York: Random House, p. 234.
Cardinali, A., & Pizzuto, A. (2011). Nuclear fusion as a massive, clean, and inexhaustible energy source for the future. Energy Science & Engineering, 1(1), 63–74. https://doi.org/10.1002/ese3.43
ITER Organization (n.d.) Advantages of fusion. Available at: https://www.iter.org/fusion-energy/advantages-fusion
Wesson, J. and Campbell, D.J. (2011) Tokamaks (Vol. 149). Oxford: Oxford University Press.
Hender, T.C., Wesley, J.C., Bialek, J., Bondeson, A., Boozer, A.H., et al. (2007) ‘MHD stability, operational limits and disruptions’, Nuclear Fusion, 47(6), pp. S128–S202. Available at: https://doi.org/10.1088/0029-5515/47/6/S03.
Seo, J., Kim, S., Jalalvand, A., et al. (2024) ‘Avoiding fusion plasma tearing instability with deep reinforcement learning’, Nature, 626, pp. 746–751. Available at: https://doi.org/10.1038/s41586-024-07024-9.
International Atomic Energy Agency (IAEA). (2023) 'Safety in fusion: Ensuring a safe path to harnessing fusion energy', IAEA Bulletin. Available at: https://www.iaea.org/bulletin/safety-in-fusion