How multilingual can a translation machine be? David Ifeoluwa Adelani from DeepMind shares new techniques to support low-resource languages, their heritage and prospects for promoting cultural diversity. By Teresa Su.
"When I moved to Germany, I couldn't understand the bills and all the documents that [were] sent to my apartment," says David Ifeoluwa Adelani, originally from Nigeria and now a DeepMind Academic Fellow at UCL. Faced with the language barrier, David turned to Google Translate but was surprised by how bad it was at Yorùbá, one of the official languages of Nigeria. Determined to improve technologies for diverse languages, he decided to combine his love for linguistics and computer science to build language models that are "underrepresented in natural language processing research where most tools do not support African languages."
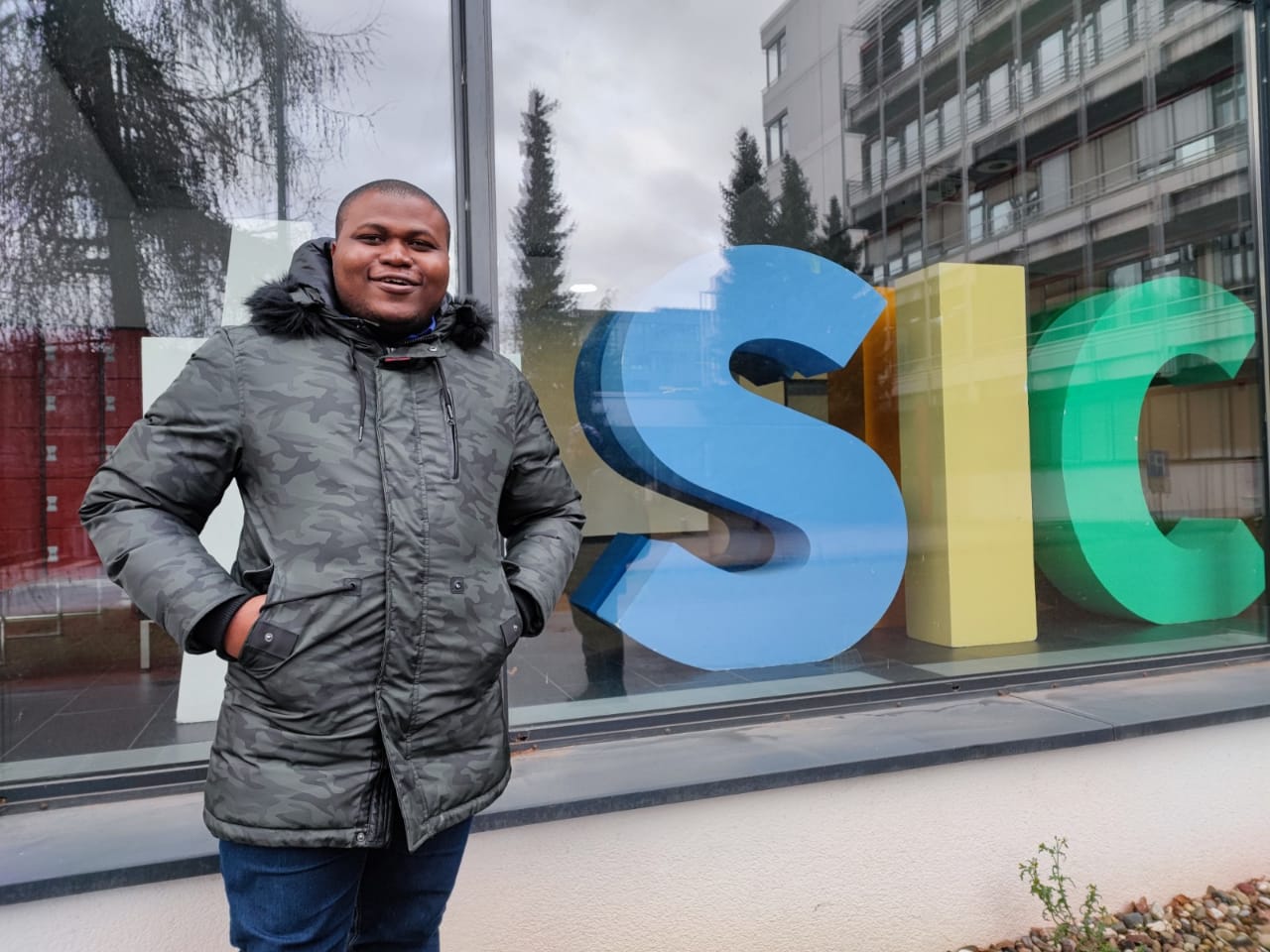
Natural language processing (NLP) is a widely integrated artificial intelligence technology that helps computers interact with humans by using languages that have evolved naturally over time. Although, its limitations arise in low-resource languages, which have relatively limited training data to help build conversational AI. For David, and many others - be they international travellers, expats or immigrants - the struggle of overcoming language barriers inevitably brings about gaps in cultural diversity.
In his latest paper [1], David and his team take multilingual pre-trained language models (PLMs) further to support relatively low-resource African languages. A pre-trained language model is analogous to a well-read human who understands the language and can be asked to perform specific tasks in that language. Some of these tasks include translation, speech recognition, natural language generation and so on. These PLMs have performed impressively well for both high- and low-resource languages, but if a language is completely unseen during pre-training, then "there is still a large performance drop..., especially [for] African languages."
An effective way of tackling this issue is language adaptive fine-tuning (LAFT), which fine-tunes a multilingual PLM on monolingual texts. Fine-tuning takes a model that has already been trained for a given task, and tweaks it to make it perform a distinct, yet similar task. However, this process can be rather inefficient as each language has to be individually adapted with the resulting model having limited cross-lingual transfer abilities. In other words, the language models that have undergone LAFT cannot be easily applied to another language without some further fine-tuning because of how specialised they are. This is like teaching Google Translate one language at a time without any improvements in its language learning skill.
So…what if we teach a model multiple languages simultaneously? Committed to improving upon this adaptive fine-tuning technique, David and his team set out to develop a multilingual adaptive fine-tuning (MAFT) system that is capable of doing exactly this: adapting a model to multiple languages at once. In their research, MAFT was performed on 17 African languages along with English, French, and Arabic simultaneously to build a single model that is highly competent at cross-lingual transfer learning for African languages.
The study [1] found that "MAFT is competitive to LAFT [and provides] a single model compared to many models specialised for individual languages.” Since language models can get very large and resource-intensive with billions of parameters, the team went further to reduce the size of the model by first removing vocabulary that don’t directly correspond to African scripts. This almost halved the size of the model, making it more lightweight for subsequent deployment and fine-tuning. Impressively, performance was not undermined by this vocabulary reduction and the model is still competitive to applying LAFT on individual languages. MAFT therefore makes it possible to have smaller models with great performance to be used on many low-resource languages. Soon, we may be able to say “Ẹ n lẹ, Siri” (“Hey, Siri” in Yorùbá). With all that Siri or any other voice assistant can do in a high-resource language such as Finnish with its 6 million native speakers, it does seem disappointing that a simple greeting in Yorùbá, the mother-tongue of almost 60 million people, is yet to be understood.
Although the development of MAFT is promising for Yorùbá and other low-resource languages speakers, there are certain challenges that are inevitable when you work at the intersection of culture and technology. For one, languages may be low-resourced precisely because their speakers aren’t active on the internet, hence the lack of digital footprint. "Because of some other previous bad experiences they have had, maybe through colonisation, people are very careful to use these technologies,” notes David. He suggests this scepticism may have risen due to the fear of valuable data being harvested from consumers and then sold back to them by big tech companies. As such, David highlights the importance "involving [users] in a process such that they feel as if this technology is built for them, not just for their own good, but also for the preservation of culture."
There is also a lack of diversity in the field of AI where most research is not concerned about under-represented communities. Therefore, David encourages students to embrace their cultural and linguistic background; "I think it's an opportunity, speaking a different language to build useful language technologies for real-life applications. It's not something to be ashamed of, it's something that you should be proud of."
References
[1]. Alabi J. O., Adelani D. I., Mosbach M., Klakow D. Adapting Pre-trained Language Models to African Languages via Multilingual Adaptive Fine-Tuning. 2022. Proceedings of the 29th International Conference on Computational Linguistics. [https://aclanthology.org/2022.coling-1.382/]